01.20.12
Posted in javascript, programming at 4:05 pm by danvk
A problem came up at work yesterday: I was creating a web page that received 64-bit hex numbers from one API. But it needed to pass them off to another API that expected decimal numbers.
Usually this would not be a problem — JavaScript has built-in functions for converting between hex and decimal:
parseInt("1234abcd", 16) = 305441741
(305441741).toString(16) = "1234abcd"
Unfortunately, for larger numbers, there’s a big problem lurking:
parseInt("123456789abcdef", 16) = 81985529216486900
(81985529216486900).toString(16) = "123456789abcdf0"
The last two digits are wrong. Why did these functions stop being inverses of one another?
The answer has to do with how JavaScript stores numbers. It uses 64-bit floating point representation for all numbers, even integers. This means that integers larger than 2^53 cannot be represented precisely. You can see this by evaluating:
(Math.pow(2, 53) + 1) - 1 = 9007199254740991
That ends with a 1, so whatever it is, it’s certainly not a power of 2. (It’s off by one).
To solve this problem, I wrote some very simple hex <-> decimal conversion functions which use arbitrary precision arithmetic. In particular, these will work for 64-bit numbers or 128-bit numbers. The code is only about 65 lines, so it’s much more lightweight than a full-fledged library for arbitrary precision arithmetic.
The algorithm is pretty cool. You can see a demo, read an explanation and get the code here:
http://danvk.org/hex2dec.html.
Permalink
01.14.12
Posted in science at 4:57 pm by danvk
I recently built a version of the CDC’s Vital Statistics database for Google’s BigQuery service. You can read more in my post on the Google Research Blog.
The Natality data set is one of the most fascinating I’ve ever worked with. It is an electronic record which goes back to 1969. Every single one of the 68 million rows in it represents a live human birth. I can’t imagine any other data set which was more… laborious… to create. :)
But beyond the data itself, the processes surrounding it also tell a fascinating story. The yearly user guides are a tour-de-force in how publishing has changed in the last forty years. The early manuals were clearly written on typewriters. To make a table, you spaced things out right, then used a ruler and a pen to draw in the lines. Desktop publishing is so easy now that it’s easy to forget how much standards have improved in the last few decades.
They’ve had to balance the statistical benefits of gathering a uniform data set year after year with a need to track a society which has evolved considerably. In 1969, your race was either “Black”, “White” or “Other”. There was a question about whether the child was “legitimate”. There were no questions about alcohol, smoking or drug use. And there was no attempt to protect privacy — most of these early records contain enough information to uniquely identify individuals (though doing so is a federal crime).
I included four example analyses on the BigQuery site. I’ll include one more here: it’s a chart of the twin rate over thirty years as a function of age.
A few takeaways from this chart:
- The twin rate is clearly a function of age.
- It used to be that older women were less likely to have twins.
- Starting around 1994, this pattern reversed itself (likely due to IVF).
- The y-axis is on a log scale, so this effect is truly dramatic.
- There has been an overall increase in the twin rate in the last thirty years.
- This increase spans all ages.
The increase in twin rate is often attributed to IVF, but the last two points indicate that this isn’t the whole story. IVF clearly has a huge effect on the twin rate for older (40+) women, but it can’t explain the increase for younger women. A 21-year old mother was 40% more likely to have twins in 2002 than she was in 1971.
My guess is that this is ultimately because of improved neonatal care. Twins pregnancies are more likely to have complications, and these are less likely to lead to miscarriages than in the past. If this interpretation is correct, then there were just as many 21-year olds pregnant with twins forty years ago. It’s just that this led to fewer births.
Chart credits: dygraphs and jQuery UI Slider.
Permalink
12.19.11
Posted in math, programming at 5:04 pm by danvk
Over the past two months, I’ve participated in Andrew Ng’s online Stanford Machine learning class. It’s a very high-level overview of the field with an emphasis on applications and techniques, rather than theory. Since I just finished the last assignment, it’s a fine time to write down my thoughts on the class!
Overall, I’ve learned quite a bit about how ML is used in practice. Some highlights for me:
- Gradient descent is a very general optimization technique. If you can calculate a function and its partial derivatives, you can use gradient descent. I was particularly impressed with the way we used it to train Neural Networks. We learned how the networks operated, but had no need to think about how to train them — we just used gradient descent.
- There are many advanced “unconstrained optimization” algorithms which can be used as alternatives to gradient descent. These often have the advantage that you don’t need to tune parameters like a learning rate.
- Regularization is used almost universally. I’d previously had very negative associations with using high-order polynomial features, since I most often saw them used in examples of overfitting. But I realize now that they are quite reasonable to add if you also make good use of regularization.
- The backpropagation algorithm for Neural Networks is really just an efficient way to compute partial derivatives (for use by gradient descent and co).
- Learning curves (plots of train/test error as a function of the number of examples) are a great way to figure out how to improve your ML algorithm. For example, if your training and test errors are both high, it means that you’re not overfitting your data set and there’s no point in gathering more data. What it does mean is that you need to add more features (e.g. the polynomial which I used to fear) in order to increase your performance.
The other takeaway is that, as in many fields, there are many “tricks of the trade” in Machine Learning. These are bits of knowledge that aren’t part of the core theory, but which are still enormously helpful for solving real-world problems.
As an example, consider the last problem in the course: Photo OCR. The problem is to take an image like this:
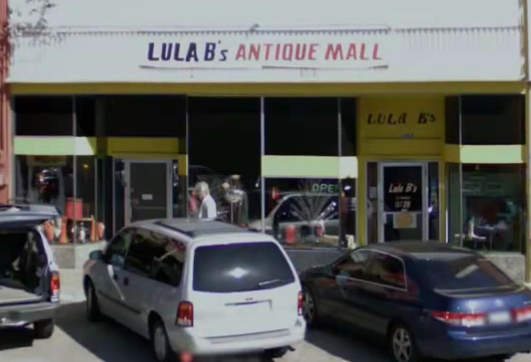
and extract all the text: “LULA B’s ANTIQUE MALL”, “LULA B’s”, “OPEN” and “Lula B’s”. Initially, this seems quite daunting. Machine Learning is clearly relevant here, but how do you break it down into concrete problems which can be attacked using ML techniques? You don’t know where the text is and you don’t even have a rough idea of the text’s size.
This is where the “tricks” come in. Binary classifiers are the “hammer” of ML. You can write a binary classifier to determine whether a fixed-size rectangle contains text:
Positive examples |
 |
Negative examples |
 |
You then run this classifier over thousands of different “windows” in the main image. This tells you where all the bits of text are. If you ignore all the non-contiguous areas, you have a pretty good sense of the bounding boxes for the text in the image.
But even given the text boxes, how do you recognize the characters? Time for another trick! We can build a binary classifier to detect a gap between letters in the center of a fixed-size rectangle:
Positive examples |
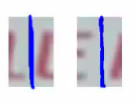 |
Negative examples |
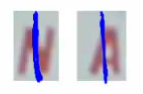 |
If we slide this along, it will tell us where each character starts and ends. So we can chop the text box up into character boxes. Once we’ve done that, classifying characters in a fixed-size rectangle is another concrete problem which can be tackled with Neural Networks or the like.
In an ML class, you’re presented with this pipeline of ML algorithms for the Photo OCR problem. It makes sense. It reduces the real-world problem into three nice clean, theoretical problems. In the class, you’d likely spend most of your time talking about those three concrete problems. In retrospect, the pipeline seems as natural as could be.
But if you were given the Photo OCR problem in the real world, you might never come up with this breakdown. Unless you knew the trick! And the only way to learn tricks like this is to see them used. And that’s my final takeaway from this practical ML class: familiarity with a vastly larger set of ML tricks.
Permalink
11.05.11
Posted in programming at 1:33 pm by danvk
It’s been almost ten years since I’ve actively used the Java programming language. In the mean time, I’ve mostly used C++. I’ve had to pick up a bit of Java again recently. Here are a few of the things that I found surprising or notable. These are all variants on “that’s changed in the last ten years” or “that’s not how C++ does it.”
The Java compiler enforces what would be conventions in C++.
For example, “public class Foo” has to be in Foo.java. In C++, this would just be a convention. You can use “private class” when you’re playing around with test code and want to use only a single file. Similarly, class foo.Bar needs to be in “foo/Bar.java”.
Java Packages are a more pervasive concept than namespaces in C++.
There’s a “default package”, but using this prevents you from loading classes by name: Class.fromName(“Foo”) won’t work, but Class.fromName(“package.Foo”) will. Classes in your current package are auto-imported, which surprised me at first. The default visibility for methods/fields in Java is “package private”, which has no analogue in C++.
Java keeps much more type information at runtime time than C++ does.
The reflection features (Class.getMethods(), Method.getParameters(), etc.) have no equivalent in C++. This leads to some seemingly-magical behaviors, e.g. naming a method “foo” in a Servlet can cause it to be served at “/foo” without you saying anything else. Not all information is kept though: you can get a list of all packages, but not a list of all classes in a package. You can request a class by its name, but you can’t get a list of all classes. You can get a list of all the method names in a class, but you can’t get a list of all the parameter names in a method.
Java enums are far richer than C/C++ enums.
enums in Java are more like classes: they can have constructors, methods, fields, even per-value method implementations. I really like this. Examples:
public enum Suit {
CLUB("C"), DIAMOND("D"), HEART("S"), SPADE("S");
private String shortName;
private Suit(shortName) { this.shortName = shortName; }
public String toString() { return shortName; }
}
Java is OK with a two-tier type system.
At its core, C++ is an attempt to put user-defined types on an equal footing with built-in types like int and char. This is in no way a goal of Java, which is quite content to have a two-tier system of primitive and non-primitive types. This means that you can’t do Map<int, int>, for instance. You have to do Map<Integer, Integer>. Autoboxing makes this less painful, but it’s still a wart in the language that you have to be aware of.
One concrete example of this is the “array[index]” notation. In C++, this is also used for maps. There’s no way to do this in Java, and I really miss it. Compare:
map[key] += 1;
to
map.put(key, 1 + map.get(key));
which has more boilerplate and is more error-prone, since you might accidentally do:
map.put(key, 1 + other_map.get(key));
The designers of Java Generics learned from the chaos of C++ templates.
Generic classes in Java are always templated on types: no more insane error messages. You can even say what interface the type has to implement. And there’s no equivalent of method specialization, a C++ feature which is often misused.
Variables/fields in Java behave more like C++ pointers than C++ values.
This is a particular gotcha for a field. For example, in C++:
class C {
public:
C() {
// foo_ is already constructed and usable here.
}
private:
Foo foo_;
};
But in Java:
class C {
public C() {
// foo is null here. We have to do foo = new Foo();
}
private Foo foo;
}
Java constructors always require a trailing (), even if they take no parameters.
This is a minor gotcha, but one I find myself running into frequently. It’s “new Foo()” instead of “new Foo” (which is acceptable in C++).
The Java foreach loop is fantastic
Compare
for (String arg : args) { ... }
to
for (Set<string>::const_iterator it = args.begin(); it != args.end(); ++it) { ... }
The “static {}” construct is nice
This lets you write code to initialize static variables. It has no clear analogue in C++. To use the Suit example above,
private static HashMap<String, Suit> name_to_suit;
static {
for (Suit s : Suit.values()) { name_to_suit.put(s.toString(), s); }
}
The new features (Generics, enums, autoboxing) that Java has gained in the last ten years make it much more pleasant to use.
Permalink
08.19.11
Posted in books, personal at 4:19 pm by danvk
I recently finished The Power Broker, Robert Caro’s critically-acclaimed biography of New York Master Builder Robert Moses. At 1200 pages, it’s an undertaking. But I’d highly recommend it if you live in the New York area.
One passage about Moses’ daily routine struck me:
A third feature of Moses’ office was his desk. It wasn’t a desk but rather a large table. The reason was simple: Moses did not like to let problems pile up. If there was one on his desk, he wanted it disposed of immediately. Similarly, when he arrived at his desk in the morning, he disposed of the stacks of mail awaiting him by calling in secretaries and going through the stacks, letter by letter, before he went on to anything else. Having a table instead of a desk was an insurance that this procedure would be followed. Since a table has no drawers, there was no place to hide papers; there was no escape from a nagging problem or a difficult-to-answer letter except to get rid of it in one way or another. And there was another advantage: when your desk was a table, you could have conferences at it without even getting up. (p. 268)
Moses’ approach to snail mail sounds a lot like the “Getting Things Done” approach to email: make your inbox a to-do list and keep it empty. Moses wouldn’t do anything until his mail was cleared. He wouldn’t let tasks pile up, so he always had a clean plate every day. He even tailored his office to enforce this workflow.
I’ve been trying the Moses technique on my work inbox recently. When I arrive in the morning, I deal with all the emails waiting for me. No excuses. No starring and leaving the message as a “to-do” in the bottom of my inbox. There are many emails/tasks that I’d prefer to ignore, but it turns out that most of them only require ten minutes of work to deal with completely.
So far, this is working well for me. But will I be able to keep it up? Robert Moses did for forty years, so there’s hope!
Permalink
« Previous Page — « Previous entries
Next entries » — Next Page »